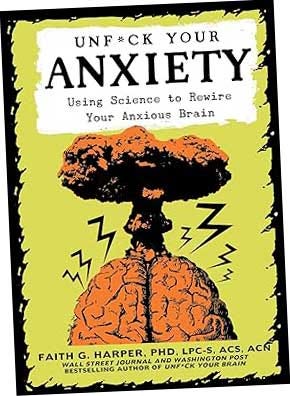
The 88-page book, Unf*ck Your Anxiety, by Dr. Faith Harper provides an overview of anxiety disorders, their symptoms, causes, and treatment options. Harper examines the underlying brain science of anxiety and its connections to chronic stress. She outlines lifestyle, medical, and complementary approaches to managing anxiety, emphasizing the importance of understanding one’s personal triggers. The book aims to empower readers to implement tools like mindfulness, cognitive reframing, nutrition, and self-care to gain control over anxiety. Harper stresses that while anxiety is challenging, it is a normal part of being human that can be unf*cked with knowledge, skills, and support.
Here are tips for what to from the book:
Practice deep breathing and progressive muscle relaxation
“The chemicals released during an anxiety or panic attack are designed to get your breathing ramped up and your heart racing.”
Slow, diaphragmatic breathing counters the physiological anxiety response. Tensing and relaxing muscle groups is deeply calming.
Engage in regular exercise or movement
“Movement, exercise, play, whatever you call it requires an engagement of attention control which takes a large enough chunk of our working memory that our anxiety rumination automatically decreases.”
Eat a nutrient-dense, whole foods diet
“The more real food you eat in general, the better for managing your health. One of the best things you can do for the entirety of your body-mind is reduce the amount of ultra-processed foods in your diet.”
Minimize processed foods and caffeine. Ensure adequate omega-3s, B vitamins, magnesium, and zinc which are commonly deficient.
Establish a mindfulness meditation practice
“Meditation releases dopamine, serotonin, oxytocin, and endorphins.”
Sitting with anxiety and observing thoughts without judgement can retrain brain pathways. Start with just 5-10 minutes daily.
What not to do
Here are some things Harper recommends against doing when trying to manage anxiety:
- Don’t avoid or try to suppress anxiety symptoms. Avoidance and suppression tend to make anxiety worse in the long run.
“Ride The Wave. Avoidance is what makes it worse. Try setting aside 5 minutes to sit with the anxiety you’re feeling instead of fighting back.”
- Don’t rely solely on medication without therapy and lifestyle changes. Medications like benzodiazepines are intended for short-term use alongside other interventions.
“They can also be habit-forming. There’s an increasing epidemic of benzodiazepine misuse.”
- Don’t self-medicate with drugs, alcohol, or other unhealthy coping mechanisms which tend to backfire.
“And speaking of Frappuccinos? Caffeine and anxiety is no bueno for very real, chemical reasons.”
- Don’t isolate yourself socially. Connecting with others provides valuable support.
“Ask for help. Because, seriously. It takes far more courage and strength to ask for and receive help than it does to give help.”
- Don’t neglect self-care like nutrition, sleep, relaxation, and exercise. Caring for your mind and body should be an ongoing priority.
“Self-care isn’t a one and done thing, it has to be a central part of our lives.”
The key is to proactively build skills to manage anxiety rather than reactively try to eliminate anxiety. Patience and lifestyle changes are essential.